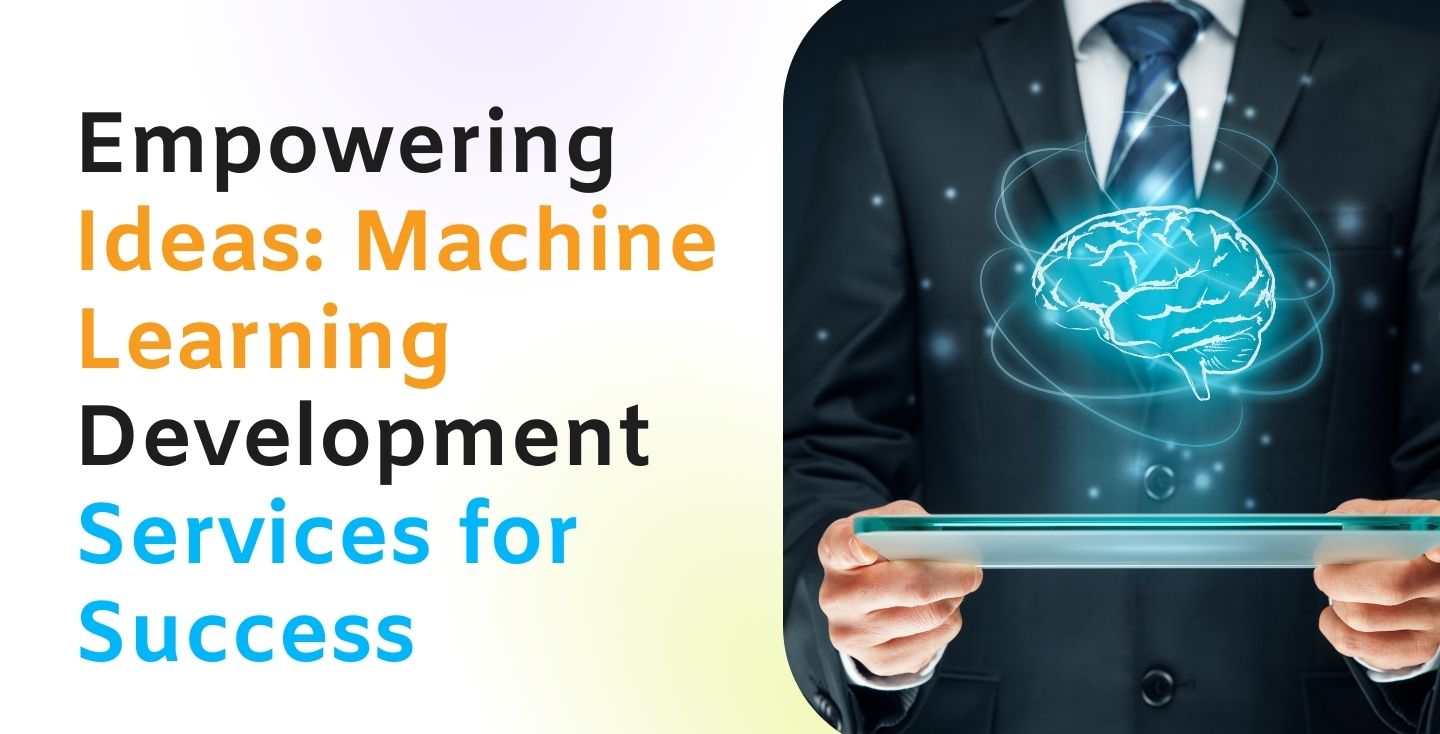
Empowering Ideas: Machine Learning Development Services for Success
Machine learning has become a revolutionary force in the ever changing and fast-paced world of technology, transforming the way businesses operate and create. Machine learning development services are in high demand as businesses try to stay ahead of the competition. This article explores the essential elements, uses, and effects of machine learning development services on corporate performance, delving into their subtleties.
I. Understanding Machine Learning Development Services
Definition and Basics
Machine learning development services involve the creation, deployment, and maintenance of machine learning models to extract insights, make predictions, and automate decision-making processes. At its core, machine learning is a subset of artificial intelligence (AI) that enables systems to learn and improve from experience without being explicitly programmed.
Types of Machine Learning Development Services
Supervised Learning: In supervised learning, the algorithm is trained on a labeled dataset, where the input and corresponding output are provided. The goal is to learn a mapping from inputs to outputs, allowing the model to make predictions on new, unseen data.
Unsupervised Learning: Unsupervised learning deals with unlabeled data, aiming to find patterns, structures, or relationships within the dataset without explicit guidance. Clustering and dimensionality reduction are common techniques in unsupervised learning.
Reinforcement Learning: Reinforcement learning involves an agent interacting with an environment and learning to make decisions by receiving feedback in the form of rewards or penalties. This approach is often used in scenarios where the model needs to make a sequence of decisions.
II. Applications of Machine Learning Development Services
1. Predictive Analytics
Machine learning development services play a crucial role in predictive analytics, allowing businesses to forecast future trends, customer behavior, and market dynamics. By analyzing historical data, machine learning models can identify patterns and make accurate predictions, enabling informed decision-making.
2. Natural Language Processing (NLP)
NLP is a field of machine learning that focuses on the interaction between computers and humans through natural language. Machine learning development services applied to NLP enable chatbots, language translation, sentiment analysis, and voice recognition, enhancing communication and user experience.
3. Computer Vision
Computer vision, powered by machine learning, enables machines to interpret and understand visual information. This has widespread applications, from facial recognition and object detection to medical image analysis and autonomous vehicles.
4. Fraud Detection
In the realm of finance and cybersecurity, machine learning development services are instrumental in detecting fraudulent activities. By analyzing transaction patterns and user behavior, models can identify anomalies and flag potential fraud, safeguarding businesses and consumers alike.
III. The Impact of Machine Learning Development Services on Business Success
1. Enhanced Decision-Making
Machine learning models process vast amounts of data at incredible speeds, providing businesses with real-time insights. This facilitates data-driven decision-making, enabling organizations to respond swiftly to market changes, customer preferences, and emerging trends.
2. Increased Efficiency and Automation
Automation is a hallmark of machine learning development services. Routine and repetitive tasks can be automated, freeing up human resources to focus on more strategic and complex aspects of business operations. This results in increased efficiency and cost-effectiveness.
3. Personalization and Customer Experience
Machine learning enables businesses to personalize products, services, and marketing strategies based on individual customer preferences and behaviors. This level of customization enhances the overall customer experience, fostering loyalty and satisfaction.
4. Competitive Advantage
Adopting machine learning development services provides a competitive edge in today’s business landscape. Organizations that harness the power of machine learning can innovate faster, optimize processes, and deliver superior products and services, positioning themselves ahead of competitors.
IV. Choosing the Right Machine Learning Development Services Provider
1. Expertise and Experience
When selecting a machine learning development services provider, it’s essential to evaluate their expertise and experience in the field. Look for a provider with a proven track record of successful projects and a team of skilled data scientists and engineers.
2. Scalability
The ability to scale machine learning solutions is crucial for accommodating growing data volumes and evolving business needs. A reliable service provider should offer scalable solutions that can adapt to the changing requirements of the business.
3. Data Security and Compliance
Given the sensitivity of data used in machine learning, security and compliance are paramount. Ensure that the service provider follows industry best practices for data security and complies with relevant regulations to mitigate risks and maintain trust.
4. Integration Capabilities
Seamless integration with existing systems and infrastructure is vital for the success of machine learning projects. Choose a provider that understands the importance of integration and can deliver solutions that work seamlessly with your organization’s ecosystem.
V. Overcoming Challenges in Machine Learning Development
1. Data Quality and Quantity
One of the primary challenges in machine learning development is ensuring the quality and quantity of data. Machine learning models heavily rely on diverse and well-curated datasets. Insufficient or poor-quality data can lead to inaccurate predictions and hinder the performance of the model.
2. Model Interpretability
As machine learning models become more complex, understanding how and why they make specific predictions can be challenging. Model interpretability is crucial, especially in industries where decisions impact individuals’ lives or involve regulatory compliance. Striking a balance between accuracy and interpretability is an ongoing challenge in the field.
3. Continuous Learning and Adaptation
In a rapidly changing environment, machine learning models must adapt to new patterns and data. Developing systems that can continuously learn and update their knowledge in real-time is a significant challenge. This requires robust algorithms and frameworks that support ongoing learning without compromising performance.
VI. Future Trends in Machine Learning Development Services
1. Explainable AI (XAI)
Addressing the challenge of model interpretability, Explainable AI (XAI) is gaining prominence. XAI focuses on developing machine learning models that provide clear and understandable explanations for their predictions, enhancing transparency and trust in AI systems.
2. Federated Learning
Federated learning is an emerging approach that enables machine learning models to be trained across multiple decentralized devices or servers holding local data. This ensures privacy and security while allowing models to benefit from the collective knowledge of diverse datasets.
3. Edge Computing and Machine Learning
The integration of edge computing and machine learning is becoming a game-changer. By deploying machine learning models directly on edge devices, such as sensors or IoT devices, organizations can achieve real-time processing, reduce latency, and conserve bandwidth.
VII. Real-World Impact of Machine Learning Development Services
1. Healthcare: Predictive Diagnostics
Machine learning has made significant strides in healthcare, particularly in predictive diagnostics. By analyzing patient data, machine learning models can predict the likelihood of diseases, enabling early intervention and personalized treatment plans.
2. Retail: Personalized Recommendations
In the retail sector, machine learning is driving personalized recommendations. E-commerce platforms leverage customer data to suggest products based on individual preferences, leading to increased sales and customer satisfaction.
3. Finance: Fraud Prevention
Financial institutions employ machine learning for fraud prevention. By analyzing transaction patterns and detecting anomalies, machine learning models can identify potential fraudulent activities in real-time, minimizing financial losses.
VIII. Ethical Considerations in Machine Learning Development
1. Bias and Fairness
Machine learning models are susceptible to biases present in training data. Addressing and mitigating bias is crucial to ensure fair and equitable outcomes, especially in applications like hiring, lending, and criminal justice.
2. Privacy Concerns
The use of personal data in machine learning raises privacy concerns. Striking a balance between extracting valuable insights and respecting individual privacy is a key ethical consideration. Compliance with data protection regulations is paramount.
3. Accountability and Transparency
As machine learning models make decisions that impact individuals and society, there is a growing need for accountability and transparency. Establishing clear guidelines and mechanisms for explaining model decisions is essential to build trust.
Conclusion
Machine learning development services are a cornerstone of innovation and success in the modern business landscape. As organizations embrace the power of machine learning, they unlock new possibilities for predictive analytics, automation, and personalized customer experiences. Choosing the right machine learning development services provider is crucial for unleashing the full potential of this transformative technology and gaining a competitive advantage in today’s dynamic market. By understanding the nuances of machine learning development services and their impact, businesses can chart a course towards a future where data-driven insights drive sustainable growth and success.